How making AI do goofy things exposes its limitations
AI-generated pickup lines, anyone?
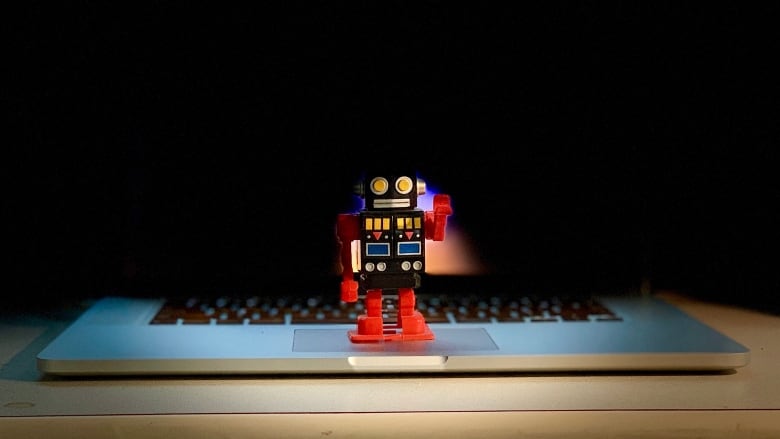
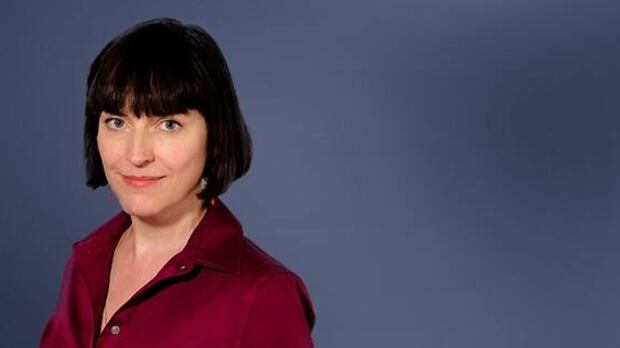
"Are you from Tennessee? Because you're the only '10' I see..."
If that makes you groan and think of an old Dudley Moore and Bo Derek movie, it's understandable.
But cheesy pick-up lines like that helped Janelle Shane expose the limitations of AI in an understandable way.
Shane is the author of You Look Like a Thing and I Love You (which is one of the lines her AI came up with), and she maintains the delightfully offbeat website, AI Weirdness.
Her new book combines funny stories about her weird experiments in pushing machine learning to the point of hilarious failure, with plain language explanations of how AI actually works.
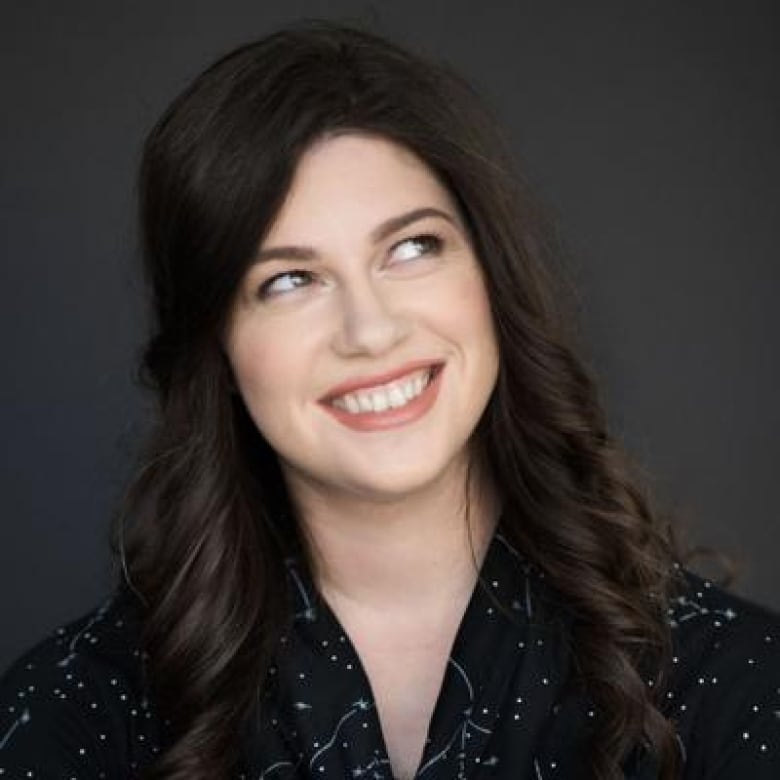
It aptly demonstrates how artificial intelligence isn't quite as intelligent as it often seems.
"Today's machine learning algorithms have somewhere around the level of an earthworm's computing power," she told Spark host Nora Young.
Which means that they are very efficient at tasks that are clearly and narrowly defined, she said, but less so at solving problems that require creativity and flexibility.
For example, chess, which has well-defined rules, is easy for an AI to master because it can play games against itself repeatedly and learn what moves work best based on statistical probabilities.
And even though most humans struggle at playing chess well, most of us can fold different types of laundry without a second thought—something that a machine-learning algorithm struggles with, because there are so many different shapes and sizes of clothing, and which often requires creativity.
"You get people trying to build "butler" bots who fold laundry and [they're] discovering, 'Oh wow, this is a harder problem than we thought'."
Shane says that it's in AI's failures that we see what it can—and can't—do. And this reveals the potential problems we need to look out for.
"I know we are seeing finally some widespread pushback against the idea that just because it's based on math an algorithm is infallible," she said.
Part of the problem stems from the way machine learning takes place: it relies on large data sets that can sometimes be incomplete, biased, or lack context.
So, for example, while a self-driving car might do well following the rules of the road, it lacks the intuition a human might have if something unusual were to happen.
"We give the algorithm this data set where it's 10,000 examples of this common thing and one of this rare thing, and it learns that it can get almost perfect accuracy just by predicting that the rare thing never happens," she said.
Other hilarious examples of Shane's experiments include asking an AI to come up with names of ice creams ("Strawberry Cream Disease," anyone?) to naming Christmas movies.
Although, who knows: Perhaps Fist of Christmas would be a smash hit.